Navigating the AI Revolution: A Guide for the C-Suite
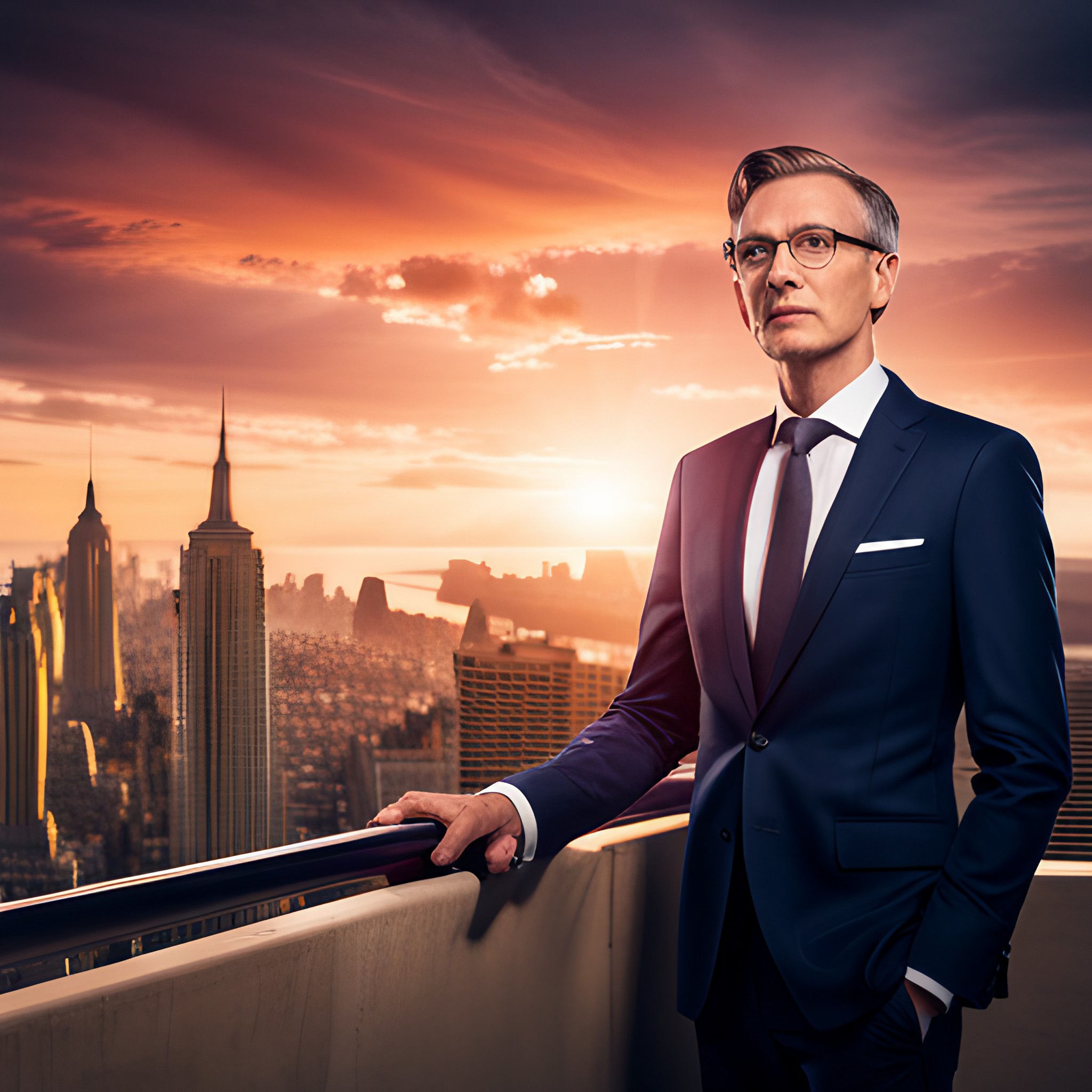
In the cacophony of buzzwords and high-tech jargon, the terms Artificial Intelligence (AI) and Machine Learning (ML) stand out. They are not just passing trends or tools of the trade for the tech-savvy. AI and ML are the co-pilots of the digital revolution, steering enterprises to unprecedented frontiers of innovation, efficiency, and sustainable growth. This article explores the imperative for C-Suite leaders to roll up their sleeves, acquaint themselves with these technologies, and lead their organizations into a future scripted by binary code and data-driven insights.
Executive Summary: Navigating the Digital Transformation with AI and ML
In today's rapidly evolving business landscape, the integration of Artificial Intelligence (AI) and Machine Learning (ML) into digital transformation strategies is not just beneficial—it's imperative for staying competitive. This article underscores the pivotal role of AI and ML technologies in redefining how businesses operate, innovate, and deliver value. Key themes include:
- Digital Transformation Initiatives: AI and ML are at the heart of digital transformation efforts, from enhancing customer experiences to optimizing supply chain management. Businesses are leveraging these technologies to develop new business models and improve efficiency across processes.
- Machine Learning Systems and Technologies: The article highlights the diverse spectrum of ML systems, from neural networks to deep learning and unsupervised machine learning, illustrating their capabilities to process and analyze vast amounts of data far beyond human intelligence capacities.
- AI’s Role in Simulating Human Intelligence: With advancements in natural language processing, computer vision, and artificial neural networks, AI systems are increasingly able to simulate aspects of human intelligence, learning from input data and making decisions with minimal human supervision.
- The Business Value of AI and ML: By implementing AI models and ML algorithms, organizations can achieve key performance indicators, gain a competitive edge, and foster innovation. Specific benefits include improved customer insights, anomaly detection in data, and more efficient business processes.
- The Challenges and Opportunities of AI Governance: The article addresses the need for robust AI governance to manage the ethical use, biases, and privacy concerns associated with AI technologies. It also discusses the importance of aligning AI initiatives with organizational culture and legacy technologies.
- Future Outlook: The promise of generative AI, artificial general intelligence, and the ongoing development of AI technologies points towards a future where the synergy between human and machine intelligence enhances every aspect of business operations.
C-Suite leaders are urged to actively champion digital transformation efforts that harness the power of AI and ML. By investing in these technologies, aligning them with business goals, and ensuring responsible governance, enterprises can unlock unprecedented opportunities for growth, innovation, and competitive advantage.
AI and ML: More Than a Tech Fad
Today, AI and ML are pivotal components of the digital transformation landscape across industries. They go beyond back-office automation and are now essential to customer-facing solutions, revolutionizing every touchpoint in the enterprise. But understanding AI and ML extends further than the boardroom's knowledge repositories; it mandates an insight into their applications and implications.
For the C-Suite, the burgeoning question is not if AI and ML, but how and where. Statistical analyses of the modern enterprise depict a tableau where data dictates strategy and AI fortifies competitive edges. Amazon's logistics optimization, Starbucks’ customer-centric AI, and Maersk’s efficient fleet management are but a few archetypes illustrating the profound impact of AI and ML integration in a digital transformation strategy. These strategies have led to cost reductions, optimized experiences, and strategic foresight that traditional business models cannot rival.
Gone are the days when intuition alone could guide the helm. In today’s landscape, decisions must be rooted in insights, with AI and ML as the essential vessels through which these insights are distilled into action. The C-Suite now faces the critical mission of integrating these technologies into the very essence of the company, beyond the periphery of ad-hoc projects and innovation labs. Only then can AI and ML evolve from mere tools to the engines propelling organizational success.
The Challenges of Adopting AI in Business
While the integration of Artificial Intelligence (AI) into business operations promises to redefine the competitive landscape, its adoption is fraught with challenges that C-Suite leaders must ingeniously navigate. The path toward AI-driven transformation is complex, requiring a nuanced understanding of technological, organizational, and ethical dimensions. Key challenges include:
- Technological Complexity: The advanced technologies underlying AI and ML systems require significant expertise to develop, deploy, and manage. Businesses often face a steep learning curve and the necessity for substantial investments in talent and infrastructure.
- Data Privacy and Security: AI systems thrive on data, raising critical concerns about privacy and security. Ensuring the protection of sensitive information while leveraging AI's capabilities is paramount, requiring robust data governance policies.
- Ethical and Bias Considerations: AI technologies can inadvertently perpetuate or amplify biases if not carefully managed. Establishing ethical guidelines and monitoring for bias in AI applications is crucial to prevent harm and ensure fairness.
- Integration with Existing Systems: Many organizations struggle with integrating AI technologies into their legacy systems. Achieving seamless integration while ensuring operational continuity presents a technical and managerial challenge.
- Cultural Resistance: Adjusting to AI-driven processes can be met with resistance from employees who fear job displacement or mistrust automated systems. Addressing these concerns and fostering a culture of innovation and continuous learning is vital for successful adoption.
- Regulatory Compliance: The fast-evolving regulatory landscape around AI poses additional complexity. Organizations must stay abreast of regulations to ensure compliance and avoid legal and reputational risks.
Navigating these challenges requires a strategic approach, with a focus on building internal capabilities, fostering partnerships, and maintaining a clear ethical compass. By addressing these hurdles head-on, businesses can fully harness the potential of AI to drive innovation and competitive advantage.
The Intricacies of Machine Learning Adoption in Business
The adoption of Machine Learning (ML) systems in business contexts is not without its complexities. These challenges stem from both the inherent technicalities of ML technologies and the broader organizational implications. Key hurdles include:
- Data Quality and Quantity: The effectiveness of ML models is heavily dependent on the availability of high-quality, relevant data. Businesses must contend with the tasks of collecting, cleansing, and labeling vast amounts of data to train these models, which can be both time-consuming and costly.
- Skill Gap: There is a pronounced skill gap in the workforce concerning ML expertise. The scarcity of skilled professionals capable of developing, deploying, and maintaining ML systems limits the pace at which businesses can adopt and leverage these technologies.
- Scalability Issues: Scaling ML projects from pilot phases to full-scale deployments across the organization involves significant challenges. It requires not just technological scalability, but also the ability to operationalize ML insights into actionable business processes.
- Model Bias and Fairness: Ensuring that ML models are unbiased and fair is a crucial challenge. Unchecked bias can lead to flawed decision-making, affecting customers and employees and potentially harming the company's reputation.
- Model Explainability: ML models, especially deep learning systems, are often seen as "black boxes" due to their complex and opaque nature. This lack of explainability can hinder trust in ML systems from both internal stakeholders and external regulators.
- Continuous Monitoring and Evolution: ML models are not set-and-forget systems. They require continuous monitoring, updating, and re-training to remain effective as the business environment and data patterns change over time.
Businesses venturing into the realm of ML must approach these challenges with a strategic mindset, investing in the necessary resources, skills, and frameworks to successfully harness the benefits of machine learning while mitigating associated risks.
Machine learning models form the core mechanism for transforming data into actionable insights, underpinning predictive analytics and intelligent decision-making. These models, powered by data-learning algorithms, identify patterns, make predictions, and optimize outcomes without specific programming for each task. Machine learning models range from simple linear regression models forecasting trends from historical data to complex deep learning networks excelling in image recognition and natural language processing. Their applicability extends across various industries and functions. The success of a machine learning model lies in its ability to adapt over time. With new data, these models can be retrained to enhance their accuracy and robustness, ensuring they remain valuable in a constantly evolving business environment. This dynamic evolution highlights the importance of machine learning models not just as static tools, but as evolving assets that adapt and grow with an organization's needs.
Understanding Neural Networks in Business Applications
The application of Neural Networks (NNs) in business settings marks a significant evolution within the broader landscape of AI and ML adoption. Neural Networks, inspired by the structure and function of the human brain, excel in identifying patterns and making predictions based on complex and voluminous data. Their multifaceted capabilities extend across various domains, from customer relationship management to fraud detection, showcasing their versatility and power within business operations. Key considerations for businesses exploring Neural Networks include:
- Complex Pattern Recognition: Neural Networks are particularly adept at recognizing intricate patterns in data, a trait that enables advanced customer segmentation, predictive analytics, and even natural language processing. This competence allows businesses to unlock deeper insights and more precisely tailored strategies.
- Adaptive Learning Abilities: NNs have the inherent capacity to learn and improve over time without being explicitly programmed for specific tasks. This adaptive learning capability ensures that businesses can keep pace with dynamic market conditions and evolving customer needs.
- Resource-Intensive Training: Despite their advantages, Neural Networks require substantial computational resources and large datasets for training. Businesses must be prepared to invest in the necessary hardware and data infrastructure to leverage NNs effectively.
- Interdisciplinary Expertise: Deploying and managing Neural Networks demands a cross-functional team that not only understands the technical intricacies of these systems but also their strategic implications within the business context. This echelon of expertise is critical for maximizing the value derived from NNs.
- Ethical and Transparency Challenges: Similar to other AI technologies, NNs face ethical considerations around bias and transparency. Efforts must be made to ensure that Neural Networks are trained on diverse datasets and that their decision-making processes are as transparent and accountable as possible.
By thoughtfully integrating Neural Networks into their operations, businesses can enhance decision-making, streamline processes, and offer more personalized customer experiences. Yet, the success of such initiatives hinges upon carefully addressing the technical, organizational, and ethical challenges associated with these advanced analytical tools.
Generative Artificial Intelligence in Business Innovation
Generative Artificial Intelligence (AI) represents a frontier in the application of AI technologies, particularly in its ability to create new content, simulate scenarios, and predict outcomes. Unlike traditional AI systems which are designed to analyze and make decisions based on existing information, generative AI models can produce novel data points, images, text, and even synthetic media that resemble human-like creations. This capability opens up unprecedented opportunities for business innovation and efficiency.
Key applications of generative AI in business contexts include:
- Content Creation: Generative AI can automate the production of marketing content, write code, generate reports, and even craft creative materials such as artwork and music, significantly reducing the time and cost involved in content development.
- Product Design and Development: By leveraging generative AI, companies can explore a vast array of design permutations quickly, accelerating the product development process and enabling more innovative solutions to emerge.
- Scenario Simulation and Forecasting: Generative models can simulate various business scenarios, from market trends to supply chain disruptions, helping organizations to prepare strategies and mitigate potential risks more effectively.
- Personalized Customer Experiences: Generative AI can enhance customer engagement by creating personalized shopping experiences, recommending products, and generating dynamic content tailored to individual preferences and behaviors.
However, the deployment of generative AI also brings challenges, such as ensuring the ethical use of generated outputs, managing intellectual property issues, and maintaining the accuracy and appropriateness of generated content. Furthermore, training these models requires access to vast datasets and significant computational power, emphasizing the need for robust infrastructure and strategic investment.
By navigating these complexities, businesses that adopt generative AI can lead in innovation, offering differentiated products and services that resonate deeply with their customers.
The Power of Data-Driven Decisions
Comparing traditional with data-driven decision-making processes illuminates the stark difference AI and ML make. Where traditional decision-making relies on historical data and subjective judgment, AI-driven approaches integrate massive amounts of data from diverse sources to predict outcomes, automate operations, and inform comprehensive strategies.
The shift to data empowerment is palpable across the gamut of industries. Predictive maintenance in manufacturing, personalized finance in banking, and dynamic pricing in retail are just the tip of the iceberg. Companies leveraging AI and ML not only outstrip their peers in efficiency and effectiveness but also in agility. Real-time data streams mean real-time strategic pivots, something executives of yore could only dream of.
Consider, for a moment, the airline industry. Where once canceled flights were an operational headache, now, through the lens of operations research and AI algorithms, airlines proactively respond to maintenance needs, crew scheduling, and even dynamic route planning based on unforeseen circumstances. It's a silent revolution being carried out in the cloud by lines of code and patterns in datasets — a revolution delivering tangible dividends on the balance sheets.
Training Data: The Foundation of Machine Learning Success
Training data is the linchpin in the development and refinement of machine learning (ML) models, including Neural Networks and Generative AI systems. This data comprises examples used to 'teach' algorithms how to process inputs, make decisions, and predict outcomes accurately. The quality, volume, and relevance of training data directly influence the performance and reliability of ML models.
A critical aspect of assembling training data is ensuring its diversity and representativeness. Models trained on narrow or biased datasets may exhibit skewed or unfair outcomes when deployed in real-world situations. Therefore, collecting a broad spectrum of data that accurately reflects the variety of scenarios an AI system may encounter is paramount.
In addition to diversity, the cleanliness and relevance of the data are vital. Preprocessing steps, including cleaning, normalizing, and labeling data, are essential to prepare a dataset for training. These tasks, while time-consuming, are crucial for avoiding the 'garbage in, garbage out' pitfall, where poor quality input data leads to ineffective AI solutions.
Furthermore, the advent of synthetic data has opened new avenues for training AI models. Synthetic data, generated by algorithms, can supplement real-world data, especially in scenarios where collecting real data is challenging, expensive, or subject to privacy concerns. This approach can also help mitigate biases in datasets by creating balanced, diverse examples that might not be available in sufficient quantities naturally.
In summary, the careful curation, preparation, and management of training data are fundamental to the success of AI projects. Businesses investing in AI technologies must prioritize establishing robust practices around training data to unlock the full potential of these powerful tools.
The C-Suite’s Role in Cultivating an AI-Ready Culture
Transitioning to an AI-powered enterprise is not solely a technological challenge; it's a cultural shift. The C-Suite must forge an ecosystem that doesn’t fear AI but rather embraces its potential while being mindful of the ethical implications.
Business leaders must craft an environment where employees are empowered to contribute to and benefit from this digital transformation. Initiatives for AI literacy and upskilling programs can level the playing field, making the promise of an AI-revolution an inclusive reality. A comprehensive Ethical AI framework is another piece in the puzzle that not only safeguards the enterprise reputation but also lays the moral foundation for AI integration.
Consider the role of chief data officers and the rise of cross-functional AI committees within organizations. These structures are not anomalies but precursors to a corporate landscape where every facet of the enterprise — every department down to the individual — is enriched by AI and ML.
Supervised vs Unsupervised Learning: Unveiling the Core of AI Training Techniques
In the realm of machine learning (ML), two primary learning paradigms stand at the forefront: supervised learning and unsupervised learning. Each approach comes with its unique methodologies, applications, and challenges, shaping the way AI systems interpret and learn from data.
Supervised Learning: The Guided Path
Supervised learning is akin to learning with a teacher. In this paradigm, the algorithm is trained on a labeled dataset, which means each piece of data comes with a corresponding label or output. The model learns to predict the output from the input data by mapping the input-output pairs during the training process. This learning method is widely used for classification tasks (where the output is a category) and regression tasks (where the output is a continuous value). Supervised learning models excel when the desired outcome of the AI system is clear, and sufficient labeled data is available to guide the learning process.
Unsupervised Learning: The Self-Discovering Journey
Contrary to supervised learning, unsupervised learning involves training an AI model on data without predefined labels. The aim is for the model to self-discover patterns, relationships, and structures within the data. Unsupervised learning techniques are pivotal in clustering and association tasks, where the goal is to segment data into groups with similar features or to uncover rules that describe large portions of the data. This form of learning is particularly valuable for exploratory data analysis, anomaly detection, and when the specific outcomes of the AI application are not known in advance.
The choice between supervised and unsupervised learning depends significantly on the nature of the problem, the goals of the AI system, and the availability of data. While supervised learning offers a more directed approach to solve problems with known outcomes, unsupervised learning offers a pathway to discovering the unknown. Together, these learning paradigms form the backbone of AI's problem-solving capabilities, driving innovation and insights across industries.
Challenges for the C-Suite in AI Integration
As organizations increasingly look to incorporate Artificial Intelligence (AI) into their operations, the C-Suite faces a myriad of challenges that extend beyond the mere technical implementation of these systems. Leadership must navigate a complex landscape of considerations to ensure successful AI integration, which includes:
- Ethical and Privacy Concerns: AI deployment raises significant ethical questions and privacy issues that organizations must address. Leadership must establish frameworks that ensure AI applications do not infringe on individuals' rights or propagate unethical practices, all while complying with global privacy regulations.
- Organizational Resistance to Change: Resistance from within can be a major barrier to the adoption of AI. The apprehension towards new technologies, fear of job displacement, and a prevailing culture of skepticism can hinder AI initiatives. The C-Suite must champion a culture shift towards openness and innovation, making the case for AI as an augmentative tool rather than a replacement.
- Budgetary Constraints and ROI Justification: Allocating budget towards AI projects, particularly those with long-term ROI horizons, can be challenging. Executives face the task of justifying these investments to stakeholders, necessitating clear articulation of the potential value and competitive advantages AI brings.
- Interdepartmental Collaboration: AI initiatives often require seamless collaboration across various departments, from IT and data science to HR and operations. The C-Suite must foster an environment that encourages cross-departmental coordination and breaks down siloed operations to fully leverage AI's capabilities.
- Keeping Pace with AI Evolution: The rapid pace at which AI technology evolves presents another significant challenge. The C-Suite must ensure the organization remains agile, with ongoing learning and development initiatives to keep skills and knowledge current, and to adapt to the latest AI advancements and best practices.
- Data Governance and Cybersecurity: With AI's heavy reliance on data, ensuring robust data governance and cybersecurity measures is paramount. Leaders must oversee the development of strategies that protect sensitive information and safeguard against data breaches, thereby maintaining customer trust and compliance with data protection laws.
Navigating these challenges requires a holistic approach, combining strategic foresight, ethical consideration, and a commitment to fostering an adaptable, AI-savvy organizational culture. The success of AI integration is contingent upon the C-Suite's ability to lead with vision, promote interdisciplinary collaboration, and uphold a firm commitment to responsible AI use.
The Ethical Dance with AI
With great power comes great responsibility. This adage from the Spiderman comics resonates poignantly in the boardrooms of today's enterprises when the discussion turns to AI.
Ethical considerations in AI development are not mere checkboxes on a project manager's todo list. They require in-depth assessments of AI systems to ensure they are trustworthy, fair, transparent, accountable, and secure. Whether it’s the de-biasing of AI models or privacy-preserving data practices, the C-Suite must champion ethical AI practices to safeguard both the reputation and the long-term viability of the enterprise.
Perhaps the most significant ethical dilemma lies on the intersection of AI and employment. Fears of job displacement must be met with resolute upskilling initiatives, ensuring that the AI wave doesn't leave a sea of unemployment in its wake. Forward-thinking companies are already investing in retraining programs, preparing their workforce for the age of AI.
Navigating Regulations in AI/ML Implementation
The regulatory landscape for Artificial Intelligence (AI) and Machine Learning (ML) is both complex and rapidly evolving, presenting yet another layer of challenge for the C-Suite. With governments and international bodies increasingly focusing on the implications of AI, a myriad of regulations are being developed to guide its ethical and safe use. These regulations aim to strike a balance between fostering innovation and protecting individual rights and societal norms.
Key Regulatory Considerations
- Global Compliance: Organizations must stay informed and compliant with a diverse range of regulations that vary by country and region. This includes understanding and adhering to the European Union's General Data Protection Regulation (GDPR), which has set precedents in data protection and privacy, and its implications for AI systems that process personal data of EU citizens.
- Transparency and Accountability: Regulations often require that AI systems be transparent and explainable. This means companies must be able to provide a clear rationale for decisions made by AI, particularly in critical areas such as credit lending, hiring, and healthcare.
- Bias and Fairness: There is a growing emphasis on creating AI systems that are free from bias and ensure fairness. This includes legal requirements to regularly audit and test AI models for biased outcomes and take corrective actions where necessary.
- Security and Safety: Ensuring the security of AI systems against cyber threats and the safety of their applications, especially in scenarios that could lead to physical harm, is a key regulatory focus. This has implications for industries ranging from automotive, where self-driving car technologies are being developed, to healthcare, where AI is being used for diagnosis and treatment planning.
Impact on Strategic Decisions
The regulatory environment requires the C-Suite to make informed strategic decisions about AI deployment, taking into account the legal, ethical, and social implications. It necessitates a proactive approach to regulatory compliance, where staying ahead of legal requirements is considered a strategic advantage.
Future Trends in AI/ML Regulation
Given the pace of technological advancement in AI and ML, we can expect a continuing evolution of regulatory frameworks. Future trends may include more standardized global regulations as international bodies seek to harmonize AI standards, and an increase in sector-specific regulations as the impact of AI becomes more pronounced in particular industries.
The responsibility of navigating this regulatory maze lies firmly with the C-Suite. Leaders must ensure that their organizations not only comply with current laws but are also prepared for future regulatory changes. Alignment with legal and ethical standards is not just about avoiding penalties; it's a strategic imperative that builds trust with customers and positions the company as a responsible leader in the age of AI.
Harnessing AI/ML for Unprecedented Innovation
The rise of Artificial Intelligence (AI) and Machine Learning (ML) marks a revolutionary era of enabling innovation, transforming industries in previously unimaginable ways. These technologies are foundational to modern innovation, driving advancements from automated customer service to predictive analytics with remarkable precision.
AI/ML goes beyond automating mundane tasks; it's about enabling innovation through new opportunities for creativity. In healthcare, AI is enabling innovation by revolutionizing diagnostics and facilitating personalized medicine. In environmental conservation, ML is enabling innovation through predictive models on climate change and optimizing resource use. In business, AI-enabled insights are transforming customer service, user experience, and operational efficiency, keeping companies competitive.
Moreover, AI/ML's role in enabling innovation extends to developing new products and services, from AI personal assistants to advanced analytics platforms, allowing companies to explore new markets and redefine their offerings. Through AI/ML, businesses are not just enhancing efficiency but are enabling innovation that tackles complex problems and meets changing consumer needs.
AI/ML's potential in enabling innovation is boundless, dependent on our ethical and responsible use of these technologies. With their vast data processing and analysis capabilities, AI and ML are paving the way for a future where innovation knows no limits, turning science fiction into reality.
Enhancing the Customer Journey with AI/ML
The advent of AI and ML technologies has ushered in a new era for customer experience, offering unparalleled opportunities to enhance the customer journey at every touchpoint. By harnessing AI and ML, businesses can now deliver personalized, seamless, and engaging experiences that not only meet but exceed customer expectations.
One of the most significant impacts of AI/ML on the customer journey is the ability to offer hyper-personalized experiences. Through the analysis of vast amounts of data, AI algorithms can understand individual customer preferences and behaviors, enabling companies to tailor their offerings, messages, and interactions to the unique needs of each customer. Personalized recommendations, dynamic content, and predictive customer service are just a few examples of how AI/ML can transform a generic customer pathway into a personalized customer adventure.
Furthermore, AI/ML can drastically improve customer service interactions. Chatbots and virtual assistants, powered by sophisticated machine learning algorithms, can provide instant assistance and support, resolving queries efficiently and freeing up human agents to handle more complex issues. These AI-driven tools can also proactively address potential concerns and offer solutions before a customer even realizes there's a problem, enhancing satisfaction and loyalty.
Predictive analytics, another powerful application of AI/ML, enables businesses to anticipate customer needs and preferences, offering products, services, and information before the customer explicitly expresses desire or intent. This forward-thinking approach can significantly enhance the customer journey, making experiences feel intuitive and thoughtfully anticipated.
By integrating AI/ML technologies into the customer experience strategy, businesses can not only streamline operations but also create a more engaging, satisfying, and memorable customer journey. The result is not just a happier customer but also a deeper and more enduring relationship between the brand and its audience.
Looking Ahead
The horizon of AI is not static but a dynamic plane continually unfurling with each technological breakthrough. Trends such as the democratization of AI, where access to these technologies is not limited to large tech companies, but to virtually every enterprise, are harbingers of a future where AI will be as ubiquitous as the internet is today.
The path to this future necessitates a strategic vision from the C-Suite, one that balances operational agility with ethical consciousness. It is a task that requires knowledge, insight, and foresight. The leaders who recognize AI and ML as the bedrock of digital transformation, not just fancy appendages, will guide their enterprises to unparalleled success.
The encroaching AI revolution is not one to be passively observed. Instead, it is a call to arms for the C-Suite, beckoning leaders to steer their organizations towards this new digital frontier. By understanding the implications, fostering a culture of innovation, and making ethical stewardship a priority, C-Suite executives will find themselves well-equipped to harness the full potential of AI and ML. The choice is clear — to be a pioneer or a plebeian in the AI age. The question is, which will your enterprise be?
In the evolving digital landscape, Artificial Intelligence (AI) and Machine Learning (ML) stand at the forefront of digital transformation, reshaping how businesses operate and driving unprecedented innovation. These technologies, leveraging vast datasets and neural networks, are not merely tools but foundational elements for creating more intelligent, efficient, and responsive systems—from natural language processing and computer vision to predictive analytics and decision-making algorithms. By simulating human intelligence, such as learning and problem-solving, AI and ML are pivotal in fostering digital transformation initiatives across various sectors. They enable the development of new business models, enhance customer experiences, and optimize supply chain management, fundamentally altering the competitive dynamics.
The integration of deep learning, a subset of ML, along with artificial neural networks, allows machines to analyze and interpret complex data structures, mirroring how the human brain works. This capacity has led to advances in fields like autonomous vehicles, anomaly detection, and generative AI, which can produce new, previously unseen content. Digital transformation strategies now prioritize the leveraging of AI and ML technologies to drive business value, improve key performance indicators, and ensure that companies remain competitive in a rapidly evolving digital economy.
Organizations are encouraged to adopt AI governance frameworks to manage the ethical use of AI and ML, facilitating a responsible approach to these powerful technologies. Data scientists play a crucial role in training these systems, employing a mix of supervised, unsupervised, and semi-supervised learning techniques to refine AI models and algorithms, ensuring they can effectively simulate human intelligence and improve business processes.
In summary, AI and ML are catalyzing digital transformation across industries, offering significant business value by enhancing operational efficiency, driving innovation, and creating more personalized customer journeys. Business leaders are called upon to strategically integrate these technologies into their operations, moving beyond legacy systems and adopting digital technologies that will shape the future of how businesses and supply chains function. In doing so, they not only meet current customer demands but also lay the groundwork for adopting future advancements, such as generative AI and artificial general intelligence, ensuring their organizations remain at the cutting edge of digital transformation efforts.
Take the Leap Forward
The digital revolution awaits no one, and the time to act is now. We invite you to join the vanguard of leaders who are already leveraging AI and ML to redefine the boundaries of what's possible in business and beyond. Don't stand on the sidelines watching the future unfold; become an active participant in shaping it. Start by evaluating where AI and ML can make the most significant impact in your operations and customer experiences today. Whether you're taking your first steps or looking to scale existing initiatives, the opportunity to innovate and lead in your industry is immense. Reach out to our team for a consultation and discover how you can harness the transformative power of AI and ML to drive your business forward. The future is in your hands; it's time to seize it.