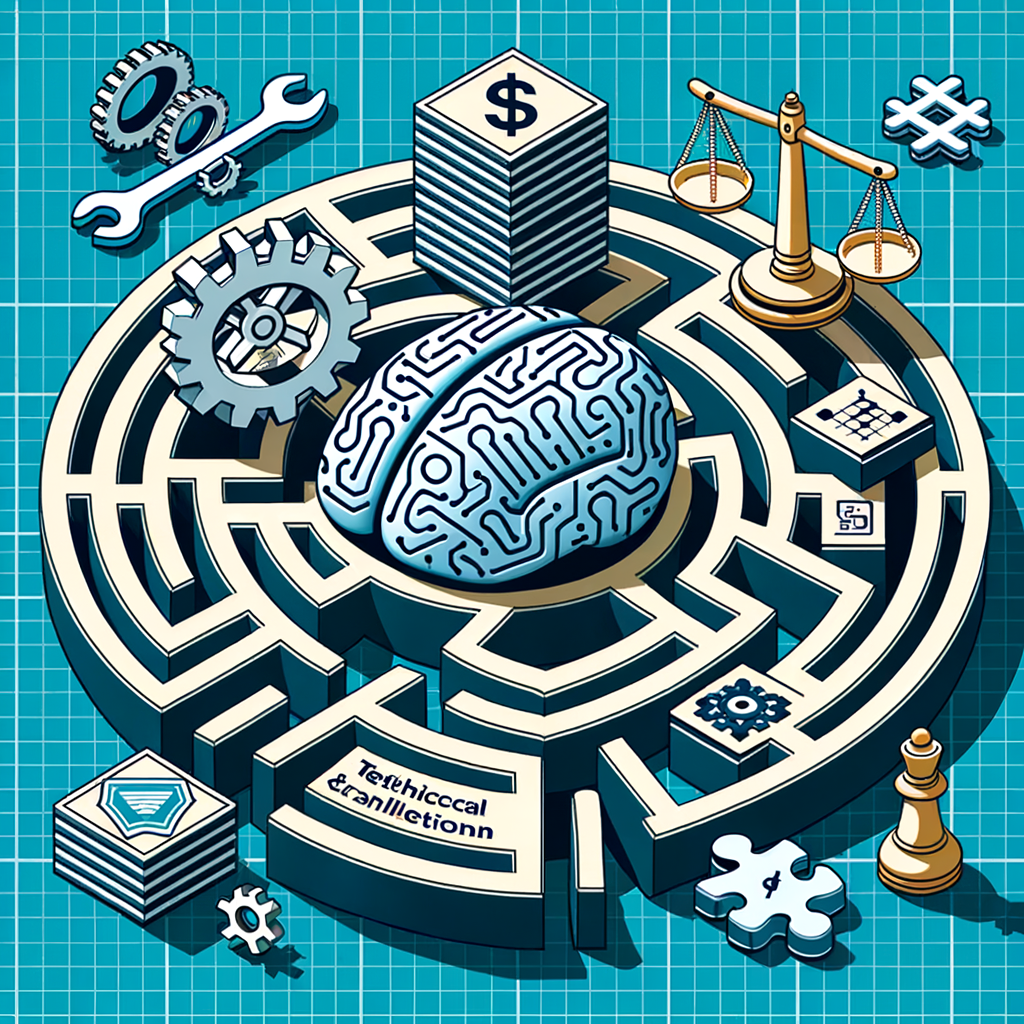
Navigating the Challenges of AI and ML Integration in the Defense Industry: Ethical, Technical, and Operational Considerations
The defense industry is a complex and ever-evolving sector, faced with a multitude of challenges that constantly shape its trajectory. One of the most pressing challenges today is the integration of advanced technologies—particularly Artificial Intelligence (AI) and Machine Learning (ML)—into defense operations. The rapid development of AI and ML comes with a host of opportunities, but also significant hurdles that defense contractors, military personnel, and policymakers must navigate.
In this article, we'll go over some of the core questions surrounding the adoption of AI and ML in defense, while exploring detailed, actionable insights. Whether you're a defense executive, engineer, or anyone involved in the industry, these questions are probably already on your radar.
What makes the defense industry different when it comes to AI and ML integration?
For starters, the stakes are much higher. In the commercial world, AI and ML are primarily used to optimize processes, enhance customer experiences, or increase profitability. However, in defense, we're talking about national security, human lives, and global stability. Mistakes in AI implementations aren’t merely costly; they can be catastrophic.
This means the defense industry has a much lower tolerance for error compared to other sectors. It also means that AI solutions must be robust, tested under extreme conditions, and able to operate in a highly dynamic battlefield environment.
Take, for example, autonomous systems like drones. In the commercial sector, a minor malfunction in a delivery drone might lead to a lost package. In defense, the loss of an autonomous UAV (unmanned aerial vehicle) due to an AI glitch could result in mission failure, or worse, unintended casualties. The standards for reliability are significantly higher.
Additionally, the defense industry has to navigate layers of bureaucracy, legacy systems, and stringent regulations when implementing new technologies. It's one thing to develop an AI algorithm in a Silicon Valley startup; it's another to make that algorithm work within the confines of military protocols and frameworks.
Are we ready for full AI autonomy in defense?
Not yet. And it depends on what we're talking about when we say "full autonomy."
In some areas, AI and ML already have a significant impact. For example, AI is being used in intelligence, surveillance, and reconnaissance (ISR) systems to process vast amounts of data faster than any human could. AI helps automate the analysis of satellite imagery, picking out potential threats and flagging them for human operators.
But when it comes to fully autonomous weapons systems, there’s still both technical and ethical hesitancy. The so-called "killer robots" debate is a major sticking point in military circles, as well as in international policy discussions. Can we entrust AI with the decision to take a human life? As of now, most nations have agreed that human oversight should remain a core element of lethal decision-making.
Let’s say you were developing an AI-driven missile defense system. While the AI might be fantastic at identifying and intercepting incoming threats, would you feel comfortable giving it the final decision on whether or not to fire? Any lapse in judgment could have severe repercussions, especially if it misidentifies a friendly aircraft as hostile.
What are the biggest technical challenges in defense AI and ML adoption?
There are a few key hurdles to address:
1. Data Quality and Availability: AI and ML algorithms thrive on data. In the commercial world, data is often abundant—think of all the data points gathered from social media, e-commerce, and consumer behavior. In defense, however, data is often classified, siloed, and not as readily available. Training AI systems requires access to this data, which can be a significant obstacle.
2. Interoperability with Legacy Systems: Many defense operations still rely on legacy systems, some of which are decades old. Integrating AI solutions into these outdated frameworks is a complex task. For example, how do you implement AI-driven threat detection into a radar system built in the 1990s? Upgrading or replacing these systems is expensive, time-consuming, and often not feasible due to budget constraints.
3. Adversarial AI: In defense, you’re not just building AI to optimize logistics or improve resource allocation—you’re up against adversaries who are actively trying to undermine your systems. Hackers and state-sponsored actors can manipulate AI by using “adversarial attacks,” which feed deliberately misleading data into the system to cause it to make mistakes. Building AI models that are resistant to such attacks is crucial but extremely challenging.
4. Ethical Considerations: As mentioned earlier, ethical concerns play a large role in the defense industry’s approach to AI. The Geneva Convention and other international laws restrict certain forms of autonomous warfare. Defense AI developers must ensure their systems follow strict ethical guidelines, which adds an extra layer of complexity to the technology.
How can the defense industry benefit from AI and ML right now?
While the road to full autonomy may have its bumps, there are several immediate benefits AI and ML can offer the defense industry today:
- Predictive Maintenance: The Department of Defense (